A World Backup Day Imperative
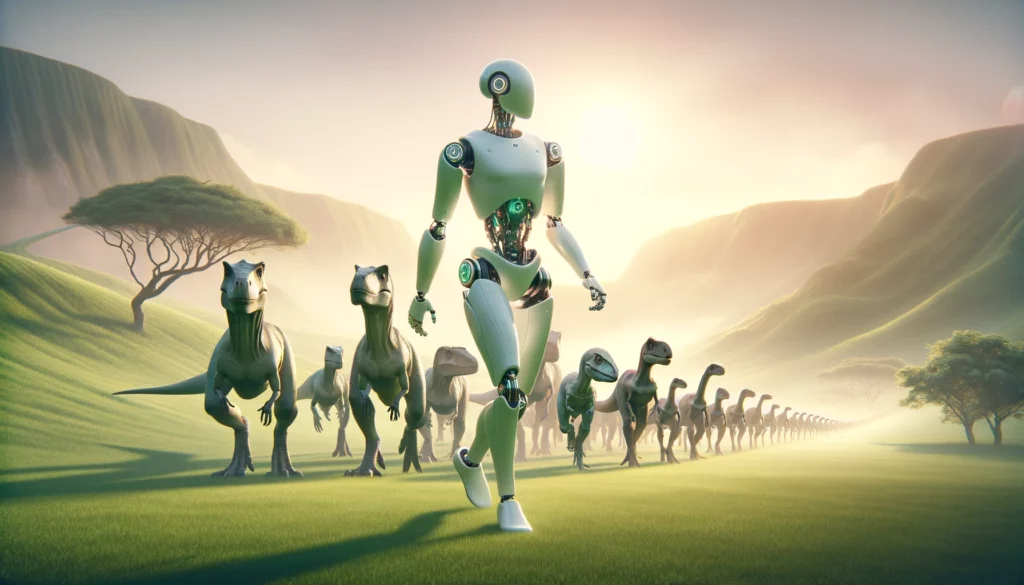
The potential for analytics and AI to transform business is undeniable. It’s why 83.9% of executives planned to increase their investments in data, analytics, and AI in 2023. But investment alone isn’t enough, as these 2023 findings from Harvard Business Review made clear:
- Only 59.5% of executives said their companies were driving business innovation with data, compared to 59.5% four years ago. That’s right – no change in four years!
- Just as worrying, only 40.8% felt they gained a competitive advantage by using data and analytics. That’s down from 47.6% four years prior.
A Yahoo Finance article points to a possible reason for this: 82% of companies say they’re making decisions based on stale information.
In an era where data acts not merely as fuel but as the very foundation upon which advancements are built, it’s clear that a better data management strategy is needed. As we celebrate World Backup Day on March 31, 2024, now is an opportune time to reflect on the importance of data management and storage in an AI-driven world.
The Need for a Robust Data Management Strategy
In simple terms, a data management strategy is a roadmap for using data to achieve your company’s goals. It helps ensure you treat data as a valuable resource by defining how activities surrounding data management—from collection to collaboration—should work together.
Data management helps organizations avoid many of the pitfalls that plague data handling. These include data silos, incomplete or duplicate data, inconsistent data quality, poorly documented data sources, and excessive vulnerability to security breaches.
A strong data management strategy ensures data is accessible, reliable, secure, and able to deliver the value you need. It makes data well-suited for analytics and AI.
Unleash your data’s value
Check out our demo to see how easy it is to go from data backup to data value.
Guidelines for creating a winning data management strategy
- Identify business objectives and requirements: Understand your organization’s goals and how data can support them. Identify the key stakeholders and their data needs.
- Assess the current data landscape: Evaluate your existing data sources, infrastructure, and processes. Identify strengths, weaknesses, opportunities, and threats.
- Define data governance framework: Establish policies, procedures, and responsibilities for managing data quality, privacy, security, and compliance.
- Data quality management: Develop processes for ensuring data accuracy, completeness, consistency, and timeliness.
- Data security and privacy: Define measures to protect sensitive data from unauthorized access, breaches, and misuse.
- Data architecture and infrastructure: Design a scalable and efficient data architecture that supports your organization’s needs. Consider factors such as data storage, integration, processing, and analytics and AI requirements.
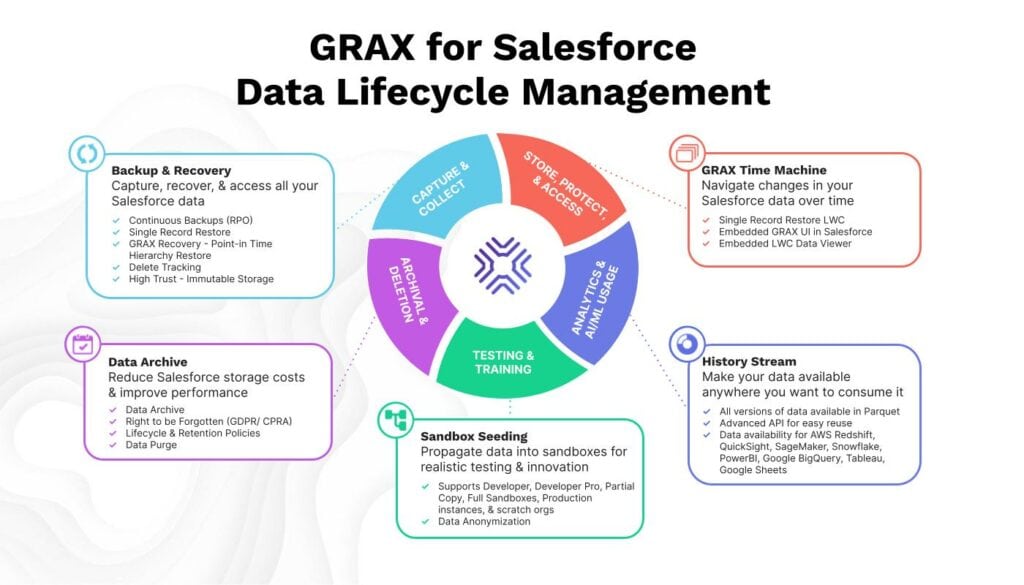
Understanding the Data Management Lifecycle
Central to any data management strategy is the data management lifecycle. It defines procedures for managing the entire lifecycle of data from acquisition to archival or disposal. You’ll also establish retention policies based on business needs and regulatory requirements such as GDPR and HIPAA.
6 phases of the data lifecycle
- Data Creation or Acquisition: Gathering data from different sources, such as Salesforce, ERP systems, and more.
- Data Maintenance: Updating and maintaining data to ensure its accuracy and relevance.
- Data Backup and Storage: Storing and protecting data so it is secure yet accessible when needed. You want to make sure all historical data is continuously backed up onto your own cloud infrastructure so that it is easy to access, restore, and use for analytics and AI.
- Data Usage: Utilizing data for decision-making, reporting, analysis, and feeding AI models.
- Data Sharing and Distribution: Making data available to stakeholders while ensuring compliance with privacy regulations and policies.
- Data Archiving and Disposal: Archiving data you don’t currently need but that may be required for compliance and other purposes. Deleting data your organization will no longer need.
Integrating the Data Analytics Lifecycle
The interplay between data management and data analytics lifecycles is where the magic happens for analytics and AI. The analytics lifecycle focuses on transforming raw data into actionable intelligence that can inform decision-making. A strategic approach to data management ensures that this data is clean, well-organized, and readily available for analysis.
7 steps of the data analytics lifecycle
- Define the problem: Identify the business problem or opportunity that analytics and AI can address
- Prepare data: Collect data, clean and prepare it for analysis
- Data exploration: Examine the data to find patterns, trends, and anomalies
- Model planning and building: Develop AI, machine learning, or other models that can analyze the data and predict outcomes
- Validate and test: Assess the model’s accuracy and effectiveness in addressing the defined problem
- Deploy: Implement the model in a real-world environment to make decisions or predictions
- Monitor: Regularly review the model’s performance and update it as necessary to adapt to new data or changing conditions
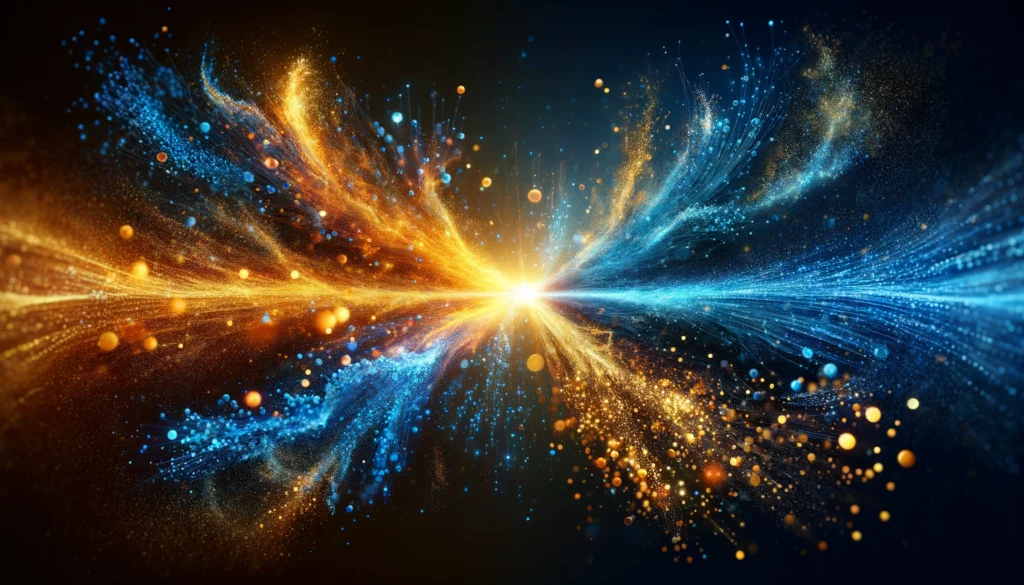
The synergy between data management and analytics
By integrating the principles of data management and analytics lifecycles, you can transform big data into a dynamic resource that drives innovation.
For example, effective data archiving can facilitate the training of machine learning models by providing access to historical data. Robust data governance ensures that the data used in analytics and AI comply with regulatory standards and ethical considerations.
5 Best Practices for Evolving Your Data Management Strategy for AI
As we mark our calendars for World Backup Day, let’s use this occasion as a catalyst to refine our data management strategies. Consider the following best practices:
- Comprehensive data governance: Review policies and frameworks that define how data is handled, ensuring compliance with regulations and ethical guidelines.
- Investment in data quality: Prioritize cleaning and validating data to enhance the accuracy of analytics and AI models.
- Scalable storage solutions: Adopt solutions that can cost-effectively accommodate your growing volume of data, ensuring it remains accessible and secure. This will likely require owning your data by storing it in your own AWS, Azure or GCP cloud infrastructure.
- Proactive backup and archiving: Regularly review and update backup and archiving practices to protect data and ensure it is available for future needs. This may include implementing more frequent backups, testing restore procedures to ensure they work as expected, making sure you have rich historical data, or revisiting data retention policies to ensure they align with the needs of analytics and AI projects.
- Cross-functional collaboration: Enhance collaboration between IT, data science, and business units to ensure data management strategies align with organizational goals and support analytics and AI initiatives.
In today’s data-driven business world, a well-conceived data management strategy isn’t a nice-to-have; it’s a must. It ensures your organization is not just prepared to navigate the complexities of an AI-enhanced digital age, but poised to thrive in it.
Ready to evolve your data strategy?
Talk to our product specialists to discover how GRAX can help