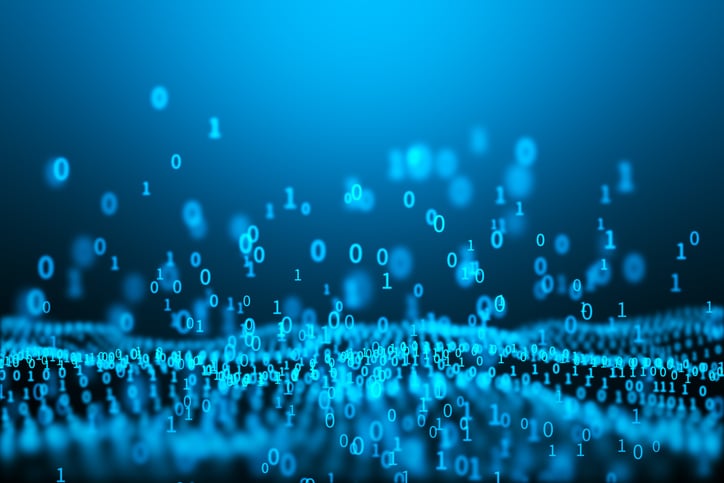
Introduction
In the modern context, information is considered the biggest asset of a company. The value that information can bring to a company is astounding, but extracting said value and using it in your decision-making process can be very challenging.
The sheer volume of data that the average company generates and collects on a regular basis brings up a lot of challenges when it comes to managing and working with said data. The aged infrastructures are no longer able to sustain business requirements, which has prompted the adoption of data lakes, data warehouses, and lately, data lakehouses.
Data lakehouses have gained immense popularity with the rapid growth of expectations from data storage and processing. The shift is rewriting data infrastructure in companies, forcing them to change their ways. Thankfully, solutions like Salesforce Data Cloud exist that can ramp up a company’s data management capabilities.
A well-configured data lakehouse will also answer modern demands around storing data and different ways of processing, all the way to performance while being future-proof for business intelligence, personalization, automation, and AI. Data lakehouses ensure cost efficiency and scalability; hence, enabling businesses to innovate and grow more without burdens from obsolete systems.
Ready to Transform Your Data into Better Insights?
Check out the GRAX Data Lakehouse in action.
Important concepts
The following section of the article will mostly cover the capabilities of Salesforce Data Cloud as a data lakehouse solution. In this context, there are multiple important terms that not all people have a clear understanding of, such as data lakes or data warehouses. Not being able to understand these two would prevent you from getting a grasp of the data lakehouse concept and the Customer 360 approach, as well. Additionally, this entire topic hinges upon the proper understanding of how structured and unstructured information differs to begin with.
How to discern unstructured data from structured data?
Unstructured data is information in multiple different forms that are difficult to compare using the same value system. This includes videos, text messages, audio logs, images, emails, and so on. This data type is usually much more difficult to sort through and analyze.
It is the original form of data that most data-gathering methods can output (which is why the overwhelming majority of information in B2B business is unstructured). Most of the unstructured data has to go through the ETL process (extract, transform, load) in order to become structured and be able to provide some sort of value to its user.
Structured data, on the other hand, is the information that has been organized already, making it significantly more readable, discoverable, and so on. The most common forms of structured data are words, strings, and numbers, and the most popular storage type for structured information is a relational database.
What is a data lake?
A data lake is the name of a modern data storage, which is built primarily for large data volumes. A data lake can store structured information, as well – although it is not the most performative solution for this data type.
Data lakes are used to store and analyze large data masses; these large volumes of information are then analyzed using dedicated software to gather valuable insights. These insights are later used to improve upon the company’s decision-making, personalization, customer support, and other departments. AI-powered analysis solutions that have become much more popular in recent years are also a significant portion of data lakes’ use cases, considering how there are little to no alternatives when it comes to storing large data volumes.
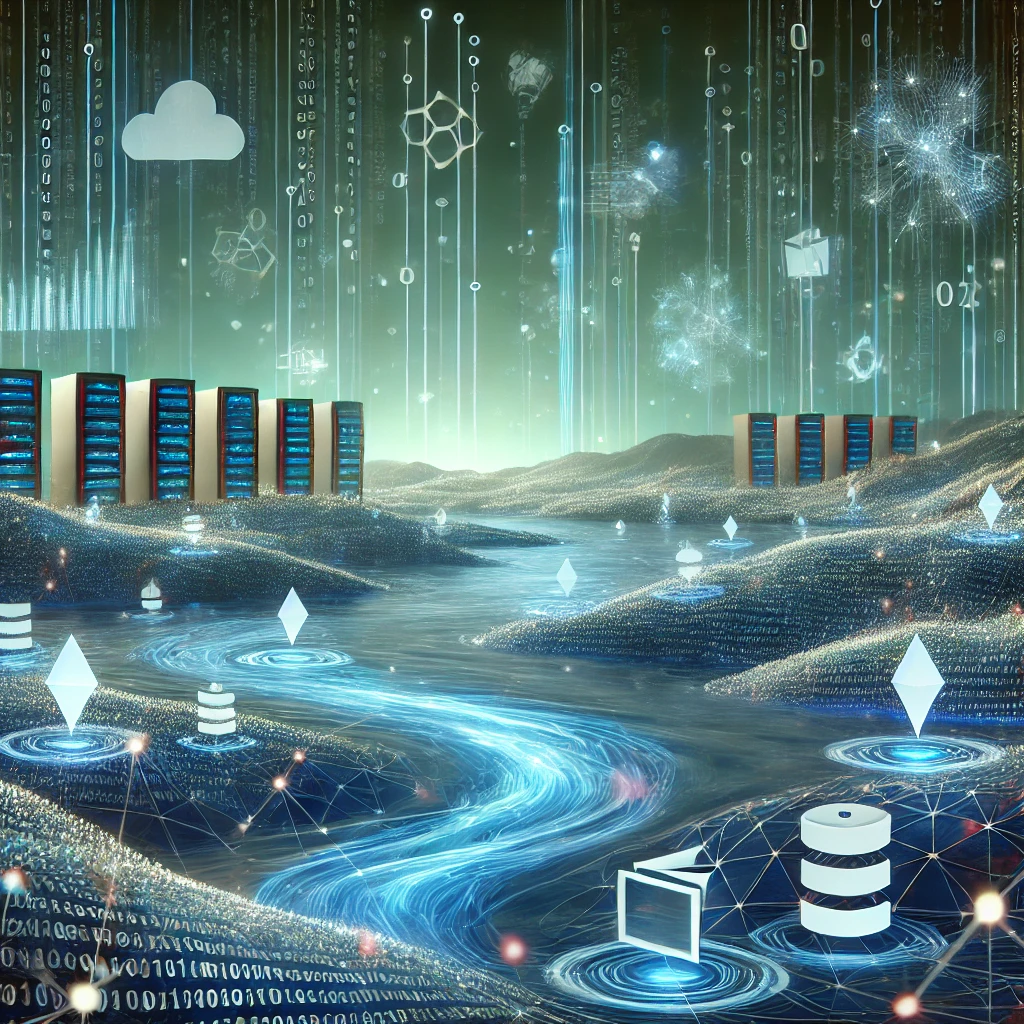
What is data warehouse?
A data warehouse acts as an antithesis to a data lake since it is a storage system built to handle processed information in a structured manner (a database). It is not uncommon for a data warehouse to be filled in a very specific order since the primary purpose of such a system is to offer extremely fast and convenient access to certain information for analysis or querying.
Data warehouses excel at handling structured information, be it business intelligence, analytical info, or historical data. At the same time, it is unfit for handling unstructured information the same way a data lake can, making it only useful in a very specific range of use cases and practically nowhere else.
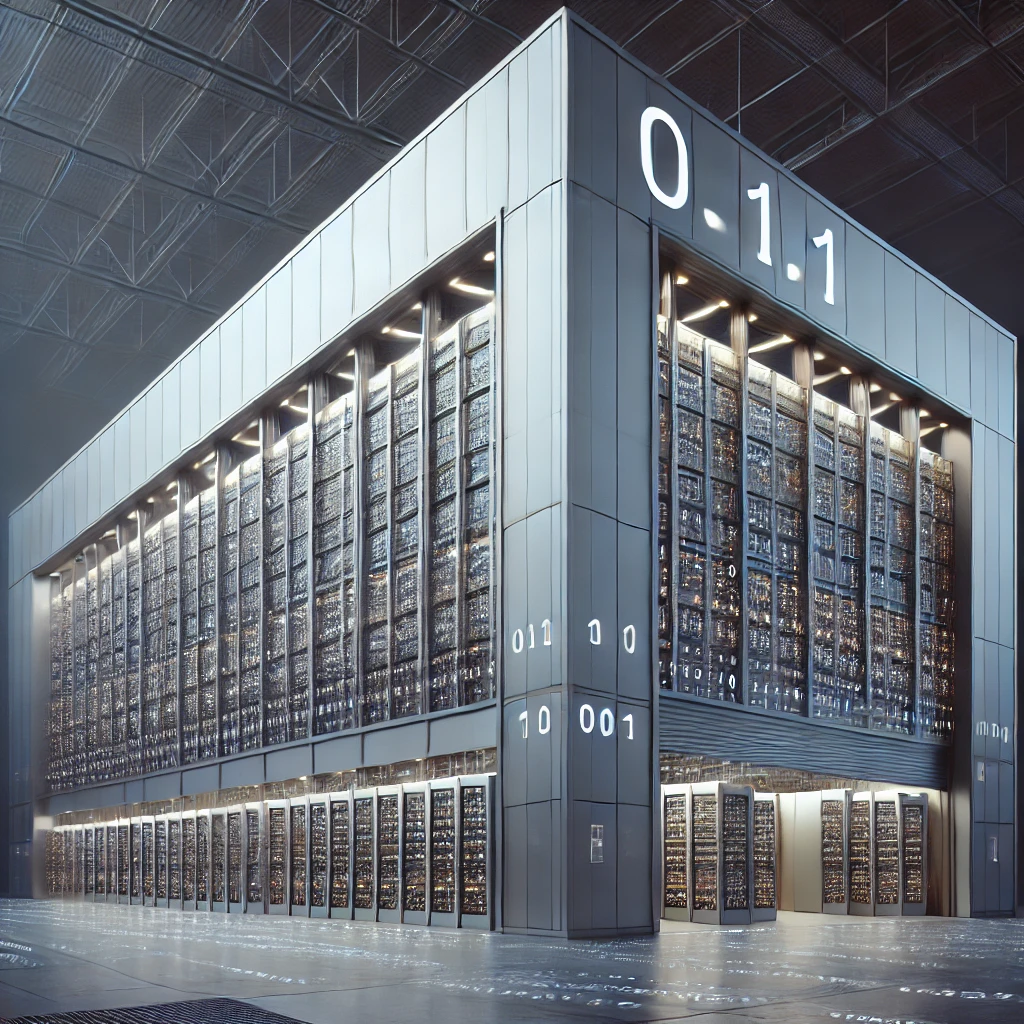
What is data lakehouse?
A data lakehouse is a more recent development in the field of data storage. It is supposed to be the combination of the two storage types mentioned above, with the benefits of both and without the disadvantages of neither. A standard data lakehouse keeps some structured elements from the data warehouse while also being able to store raw data with the same efficiency as a data lake.
The most prominent example of a data lakehouse is Salesforce Data Cloud – a versatile data platform that can offer multiple ways to store information from external sources while also generating insights, improving workflows, and so on. Data Cloud also manages to highlight the most notable use cases for data lakehouses – information storage for machine learning, analytics, and general data exploration.
Being able to drastically reduce the separation between data lakes and data warehouses remains the most notable feature of a data lakehouse. It manages to offer the incredible speed of structure querying in combination with the efficiency of storing large data masses. At the same time, the sheer complexity of a data lakehouse is significantly higher than that of a data lake or a data warehouse; it is both difficult to implement and quite challenging to manage afterward.
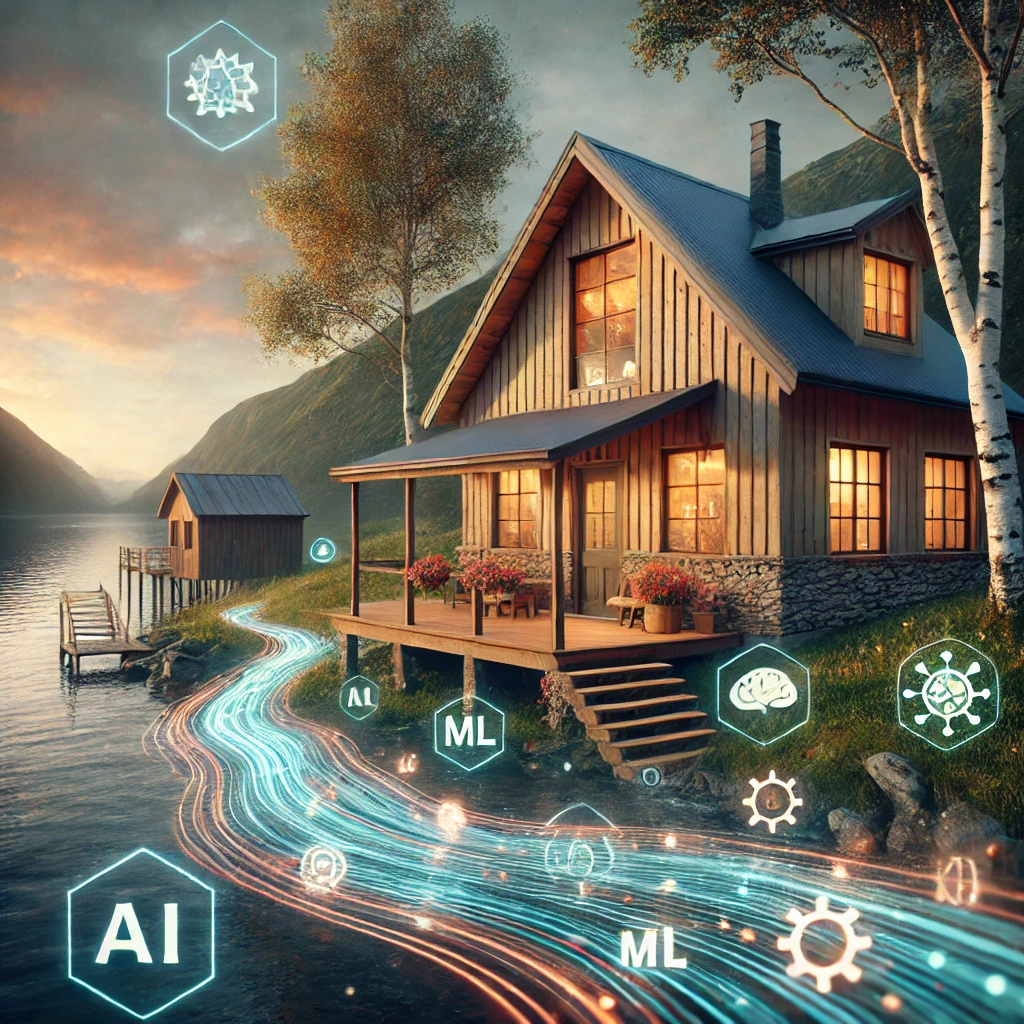
Comparison table for data lakes, warehouses, and lakehouses
To simplify the comparison between the three data management approaches, we have prepared a simple table that explains the basic advantages, shortcomings, and capabilities of each option:
Benefits | Shortcomings | Use cases | Data types | Storage type | |
Data Lake | Versatility, compatibility with different data types | Problematic governance, varying data quality | Primary storage, basic analysis | Unprocessed (raw) data | No schema |
Data Warehouse | Impressive performance, outstanding data consistency | Very basic flexibility, not suitable for handling raw data | Business intelligence, reporting | Processed data organized in a specific structure | Rigid pre-set schema |
Data Lakehouse | Structured querying with surprising performance | Complicated management and resource-heavy implementation | Machine learning, AI-assisted analytics in real time | Both raw and processed data | No schema with a few structured elements |
What is Customer 360?
Customer 360 is the approach to customer information looking at every single touchpoint and interaction as the means of creating a comprehensive overview for the customer in question.
Each customer profile is created by aggregating and converting information (preferences, behavior patterns, demographics, purchase history, and many others) from multiple sources – customer service, marketing, sales, social media, etc. Customer 360 was created to offer businesses a deeper understanding of their customers to open up more opportunities for personalization.
Salesforce is one of the biggest contributors to the Customer 360 model, and its Data Cloud solution manages to integrate information from multiple sources into a single comprehensive overview of a customer. The advantages of such an approach are rather obvious: better customer retention, higher marketing efficiency, improved customer service, and so on.
Unlock Customer Insights Faster
Discover how a GRAX data lakehouse can help.
Data management in Salesforce
Salesforce managed to completely revolutionize the entire field in its early days. It is a cloud-based solution that has been offering comprehensive customer interaction management with marketing, sales and service management on one platform.
The traditional CRM system relied a lot on structured information, such as leads, accounts, contacts, opportunities, etc. However, the interactions between companies and customers have become more and more complex with time, making traditional CRM less effective with each passing year. As such, the necessity for dynamic information storage with real-time insights was born.
As businesses became more focused on collecting unstructured information from emails, devices, and social media pages, Salesforce had to improve itself to remain competitive. This is how the Salesforce Data Cloud was born, along with the Customer 360 approach.
Salesforce Data Cloud
The introduction of a brand-new data storage solution made it possible for Salesforce to stay on top of the data management market, making it possible for businesses to aggregate and analyze massive data volumes with no penalties when it comes to performance or storage space. The solution in question is Salesforce Data Cloud – and its completely revolutionary data lakehouse approach.
Salesforce Data Cloud is a representation of how far the company has come in helping businesses with data management and data-driven decisions. It is a real-time data management platform with excellent scalability; it can support both structured and unstructured data, and its dedication to the Customer 360 approach makes it significantly easier for businesses to receive the full potential of the information they gather.
The data lakehouse concept is one of the fundamental advantages of Salesforce Data Cloud, offering the benefits of a data lake and a data warehouse in a single package. Being able to provide the scalability and flexibility of a lake together with the performance of a warehouse makes Salesforce Data Cloud an excellent customer data storage solution that can work with any data type without significant performance losses.

Benefits and unusual capabilities of Salesforce Data Cloud
Most of the advantages of a data lakehouse as a concept are also applicable to the Salesforce Data Cloud, including:
- Unified architecture results in the advantages of both approaches to data storage, along with several other advantages as a bonus.
- Extensive analytics, with support for batch processing and real-time processing, offers the flexibility of multiple data analysis methods to its users. The structure of the Data Cloud is also flexible enough to support the more demanding types of analytical tasks – including AI- and ML-powered capabilities.
- Impressive scalability and the ability to work with different data types in the same environment, no matter if the data itself is structured or not.
- Enhanced data governance workflows, with a larger emphasis on information security and plenty of data governance capabilities – data lineage tracking, audit logs, precise access control, and so on.
- Improved cost efficiency, resulting in low-cost storage being used for all storage tasks while also allowing for extensive data processing to happen. The ability to analyze the data itself also drastically reduces the necessity to move information for a task as simple as data analysis.
However, these advantages are somewhat generic and do not describe the depth and expertise of Data Cloud’s capabilities. As such, we can also present two unique features that only Salesforce’s solution has:
- The star schema is a dedicated data modeling technique that drastically simplifies querying processes by organizing information into fact and dimension tables. It excels at working with large data sets, allowing for a quick and easy extraction of valuable insights.
- The simplified ETL (extract, transmit, load) process is also a noteworthy advantage of Salesforce Data Cloud, making it possible to streamline the majority of the data movement, making the analysis process a lot faster and more efficient.
Use cases and applications for data lakehouses
There are four primary categories of use cases that data lakehouses such as Salesforce Data Cloud excel at:
Personalized marketing
Personalized marketing campaigns are brought to an absolutely new level with the introduction of data lakehouses. Being able to store and analyze large data masses from multiple sources offers the ability to create an in-depth profile for each customer. These profiles make the creation of highly targeted marketing campaigns significantly easier, which is a great option for individual high-value clients.
The most common example of such personalization is behavioral pattern discovery using information from data lakehouses, which makes it possible to create personalized advertisements and email campaigns based on the specific needs and pain points of a specific customer. Personalization itself is considered a substantial advantage in the entire marketing field, boosting engagement, driving better ROIs, and improving conversion rates.
Customer support
Data lakehouses can also prove themselves highly advantageous when it comes to improving customer support experience across the board. A data lakehouse makes it possible for a customer support member to have a complete overview of the entire history of inquiries and purchase records within the company, making each interaction far more individualized. The ability to thoroughly analyze information also enables data lakehouses to discover common issues and try to address them proactively, potentially preventing a number of similar issues in the future.
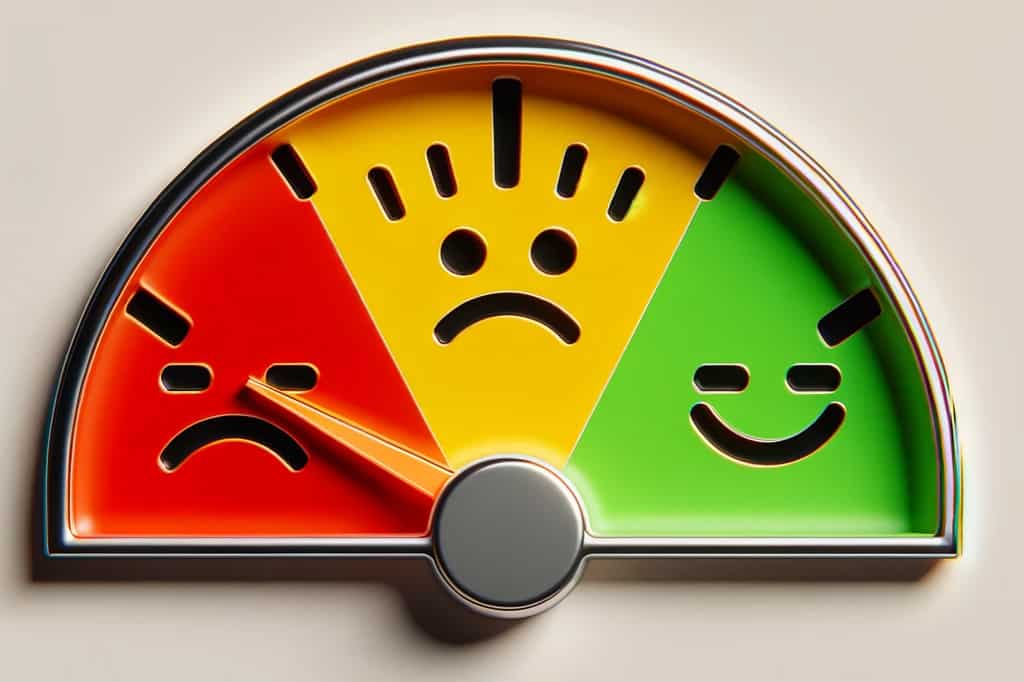
Recommendations
The same level of information richness makes it possible for data lakehouses to contribute significantly toward product recommendations to end users. These recommendations can get on a whole other level if the system itself has access to past purchases, preferences, and browsing history, making it a lot easier to address the specific requirements of each customer. Retail and e-commerce fields are where this feature shines, often resulting in significant sales improvements, along with other advantages.
Data-driven decision-making
Data-driven decision-making is the last and probably the most important application for data lakehouses. Information centralization makes it possible for all departments of the company to work with the same information, reducing the number of inaccuracies and removing the potential for information mismatch. Information consistency and accuracy can improve the majority of performance metrics of a company at once. Every single department of a company works with data in some way – sales teams refine strategies in accordance with customer trends, finance teams optimize budgets, and so on.
Implementing a data lakehouse solution
The topic of implementing a data lakehouse solution is relatively similar for most variations of the software available, but there are some specific elements that are going to be easier to work with, specifically in the case of Salesforce Data Cloud.
Essentially, there are three important factors that are worth going over when it comes to data lakehouse implementation: data cleansing, integration, and security.
Data cleansing is a preparation step that is necessary to perform before implementing a data lakehouse. The primary purpose of this action is to ensure that the information you already have is error-free, accurate, and consistent – all of which is important in the context of sheer data volumes that data lakehouses operate with.
Other features of the data cleansing process include format standardization, duplicate detection (and removal), and a number of other procedures that are needed to ensure the information is ready for further processing.
Integration with existing environments and storage infrastructures is another borderline essential element of the preparation process. Most companies tend to store their information using several different storage systems, with a frequent inclusion of legacy software or hardware. Being able to integrate all of these systems to work correctly with the data lakehouse can be moderately challenging.
The most common examples of necessary integration steps are direct connections with data sources, real-time data ingestion pipelines, and even something as simple as software compatibility checks with data management solutions. Proper integration of a data lakehouse solution with the organization’s storage system offers a multitude of advantages, such as a drastic reduction in the number of data silos, centralized access to all of the company’s information at once, and a lot of potential for comprehensive data analysis.
The topic of security (and compliance) is extremely important in this context, considering how much of the customer information can be considered sensitive and protected with various government regulations. Plenty of safety considerations must be implemented in order to secure this information, with features such as access controls, regular audits, encryption, and more.
Compliance is just as important here, including both data protection laws and industry regulations, so verifying what kind of regulations your data falls under would be the first step. In most cases, a data governance framework of sorts has to be implemented, making sure that the information is stored and processed in accordance with all the legal requirements while having discoverable audit trails for further usage.
Salesforce Data Lake and Lakehouse solutions from GRAX
Of course, the actual implementation difficulty also differs significantly depending on the solution itself. For example, GRAX has two of the most potent solutions for Salesforce data management: GRAX Data Lake and GRAX Data Lakehouse.
GRAX Data Lake is a flexible and secure storage of Salesforce data in any format. With this storage, organizations are able to retain access and analyze historical data. It provides high availability for deep analytics and offers a Customer 360-degree view for a holistic understanding of the customers.
GRAX Data Lakehouse is an intelligent, extremely capable data management platform that brings together the strengths of data lakes and warehouses. One-click deploy, it provides better performance for querying and searching data. It extracts valuable insights from a sea of diverse data and provides the scalability and performance required by today’s modern data-driven decision-making. It is a future-proof platform solution that supports enterprise analytics, AI, and machine learning requirements of the businesses that are increasingly growing in demand.
These solutions will help your enterprise keep its Salesforce data in better shape while pushing deep insights and operational efficiency.
Solve Your Data Challenges, Answer Business Questions.
Take the first step to better data-driven decision-making. Talk with a product expert today.
Future Trends and Conclusions
It is completely natural to expect a technology such as data lakehouse to keep evolving as time goes on, introducing more features and improving the existing ones. Two of the most notable developments in the tech market right now are machine learning and AI, so their integration with the Salesforce solution is going to continue developing to be able to offer better insights, more automation, better performance and accuracy, and so on.
That’s not to say that the software itself would not evolve, as well. User interface improvements, better integration with third-party storage, and improved data visualization are just a few examples of how Salesforce Data Cloud and other similar solutions based on the concept of a data lakehouse are going to improve as time goes on.
Data lakehouses offer a practically irreplaceable solution to plenty of issues that the companies have been dealing with for several years now. In this context, the importance of solutions such as Salesforce Data Cloud is very difficult to overestimate, considering how they can offer incredible data management and data analysis capabilities in a single versatile solution.
Data lakehouses can provide a comprehensive overview of the company’s customer base, drastically improving the company’s capabilities to perform relevant data-driven decisions. Such a technology can also offer plenty of potential when it comes to capitalizing on various opportunities and future trends when necessary. It is highly recommended to start using the data lakehouse technology as early as possible, especially for companies that are already working with an iteration of a data lake or a data warehouse.